Unlocking Value Through Image Segmentation ML in Business
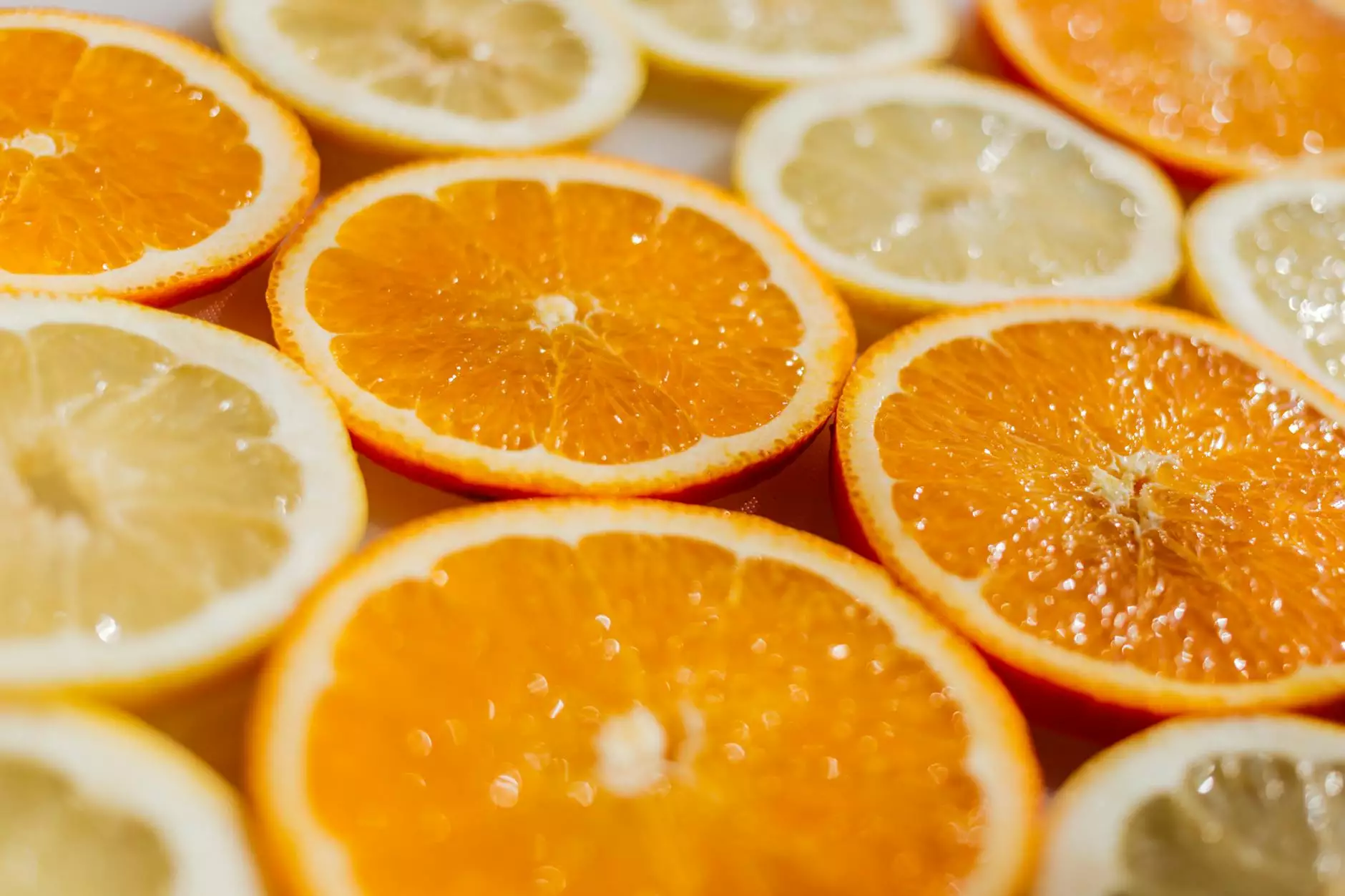
Understanding Image Segmentation
Image segmentation refers to the process of partitioning an image into multiple segments or regions, making it easier to analyze in depth. The power of this technique is magnified when combined with machine learning (ML), which allows for automated, intelligent analysis of visual data. This intersection of image segmentation and machine learning is transforming various industries, providing businesses with actionable insights and a competitive advantage.
The Relevance of Image Segmentation in Business
With the rise of digital platforms and the increasing amount of visual data generated daily, businesses can no longer afford to overlook the importance of image segmentation ML. This technology enables businesses to understand consumer behavior, optimize operations, and implement targeted marketing strategies. Let's delve deeper into the impact and application of image segmentation across various sectors.
1. Enhancing Marketing Strategies
In the realm of marketing, understanding customer preferences is paramount. By employing image segmentation ML, businesses can analyze images from social media, advertisement responses, and customer-generated content to decipher what resonates with their target audience.
- Targeted Advertising: Businesses can refine their advertising by identifying which images attract the most engagement from specific demographics.
- Sentiment Analysis: Analyzing customer-submitted images allows for a better understanding of sentiment associated with products or services.
2. Optimizing Supply Chain Operations
Efficient supply chain management is crucial for any business. Integrating image segmentation ML into logistics can lead to significant enhancements. For instance:
- Inventory Management: Automated analysis of images in warehouses can help track stock levels and reorder supplies promptly.
- Quality Control: Images of products can be assessed for defects or variances from quality standards.
3. Revolutionizing Customer Service
Picture this: a customer contacts support with an issue related to a product. Using image segmentation ML, customer service representatives can quickly analyze the image the customer provides, allowing for faster resolutions.
Implementing Image Segmentation ML
Transitioning to an image segmentation ML strategy involves several key steps. Here’s a detailed approach businesses can follow:
- Data Collection: Gather a comprehensive dataset of images that represent the business's operational aspects, customer interactions, and product offerings.
- Preprocessing: Clean and prepare the images for analysis, ensuring the data is consistent and well-labeled.
- Model Selection: Choose a machine learning model best suited for the segmentation task, such as Convolutional Neural Networks (CNNs).
- Training: Train the model using the segmented images to ensure accurate learning and recognition capabilities.
- Testing and Validation: Rigorously test the model’s performance with new data to ensure reliability and accuracy.
- Deployment: Implement the trained model into business operations, continuously monitoring its performance and making adjustments as needed.
Case Studies of Successful Implementation
1. E-commerce Platforms
E-commerce giants are leveraging image segmentation ML to enhance shopper experiences. By analyzing product images, these companies can identify which attributes—like color, shape, or style—are most appealing to their consumers. This granularity in understanding helps personalize the shopping experience, leading to increased customer satisfaction and retention.
2. Healthcare Applications
In the medical field, image segmentation is critical. Innovations in image segmentation ML have facilitated the accurate diagnosis of diseases through medical imaging techniques. For example, detecting tumors in MRI or CT scans is significantly enhanced through precise segmentation, which can lead to timely intervention and treatment.
Challenges and Considerations
While image segmentation ML offers numerous benefits, businesses must also navigate certain challenges:
- Data Quality: High-quality, well-annotated data is essential for the success of machine learning models.
- Resource Intensive: Initial implementation and training processes can be resource-heavy, requiring significant time and expertise.
- Integration: Seamlessly integrating this technology into existing systems can present operational challenges.
Future of Image Segmentation in Business
The future is bright for the application of image segmentation ML in business. As technology advances, we can expect:
- Improved Accuracy: More sophisticated models will result in enhanced accuracy in segmentation tasks.
- Broader Applications: Industries like agriculture and environmental monitoring will also tap into segmentation technology.
- Real-time Processing: With advancements in computational power, real-time image analysis will become a reality, allowing for immediate business insights.
Conclusion: Invest in the Future with Image Segmentation ML
In the ever-evolving landscape of business, leveraging advanced technologies like image segmentation ML is no longer a luxury but a necessity. From optimizing marketing strategies to enhancing customer service and streamlining operations, the applications are limitless. By investing in this technology, businesses can unlock tremendous value, drive efficiency, and position themselves ahead of their competitors. Embracing image segmentation ML today sets the foundation for a more insightful, responsive, and successful tomorrow.
Get Started with Keymakr
If you're looking to integrate advanced technologies within your business operations, Keymakr offers extensive resources and support in the realms of home services, keys, and locksmiths. Explore how innovative solutions can streamline your processes and enhance your customer relations.